Artificial Intelligence and What it Means for the Rail Industry
Written by Matthew Dick, P.E.,Chief of Strategy & Development, TTC Operated by ENSCO, Pueblo, Colo.
RAVENSWORTH, Va. –– FROM THE MARCH 2024 ISSUE OF RAILWAY TRACK AND STRUCTURES –– TTC Operated by Ensco.
Matthew Dick, P.E., Chief of Strategy & Development, ENSCO, Inc., Pueblo, CO
Serkan Sandikcioglu, AI ML Business Area Lead, ENSCO, Inc., Charlottesville, VA
Artificial Intelligence (AI) has recently experienced a significant leap forward. AI, a broad term encompassing software tasked with performing various functions, may either replicate tasks historically done by humans or pioneer new territories. Machine Learning (ML) is closely intertwined with AI, employing software and mathematical techniques to process analytical data, with “algorithms” being a primary function of ML. This article will delve into what AI entails and its implications for the rail industry.
What are Algorithms?
Machine Learning, existing for decades, manifests in various forms, with neural networks currently at the forefront. Operating akin to human brains, neural networks necessitate training data with defined inputs and outputs. For instance, a dataset featuring animal photographs labeled as “cat,” “dog,” “goat,” etc., serves as training data. Both human children and neural networks learn by associating images with their labels. Once trained, the model identifies the type of animal in unseen pictures.
However, there are a few important items to keep in mind. Firstly, neural networks achieve higher accuracy with extensive training data. Secondly, they struggle when tasked beyond their training data parameters. For example, if presented with a photograph of an elephant, the algorithm would struggle as elephants weren’t part of the training data. Thus, broad, and exhaustive training data is imperative for optimal performance.
One of the leading AI companies, OpenAI, developed ChatGPT, a Large Language Model (LLM) specializing in generating written text from prompts. Prompts are the request to the AI tool that triggers it to create an output. For example, giving ChatGPT the prompt “Please generate a checklist of safety inspection items that should be inspected for on a hi-rail vehicle”, will result in ChatGPT generating an impressive, but maybe not 100% accurate hi-rail safety inspection checklist. Prompt Engineering refers to best practice of effective prompts. Apart from ChatGPT, other LLMs like Microsoft’s Azure, Google’s Bard, and Meta’s Llama, alongside text-to-image and text-to-video AI tools such as Midjourney, DALL-E, Stable Diffusion, and Sora, have gained popularity for their impressive outputs.
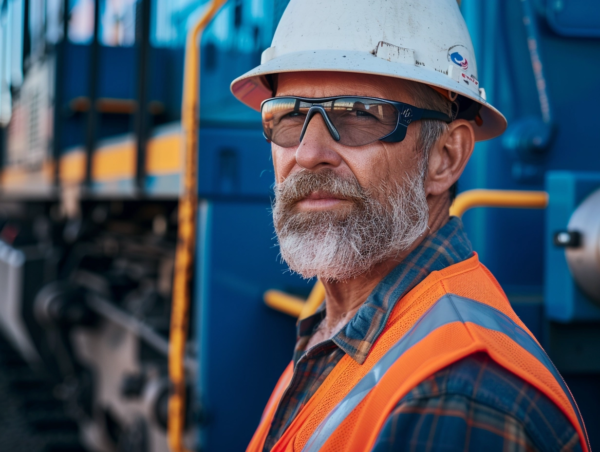
Figure 1. Example AI generated image using Midjourney v6.
Why is AI Big Now?
Machine learning, neural networks, and algorithms have all been in existence for decades. Why is AI now seemingly skyrocketing, with new companies and tools emerging each month? Several culminating factors are at play, enabling today’s expanding AI.
Firstly, there are astronomically large training datasets. The amount of written text, images, and videos available on the internet has exploded over the past few decades, with social media being one of the largest drivers of this growth. All the AI tools mentioned above utilize vast amounts of data obtained from the internet via public sources and licensed use.
The second factor is the openly shared ML techniques. An interesting aspect of the growth of machine learning is its roots in academic research, where openly sharing research results is the norm through published papers and open-source software. This has dramatically accelerated the evolution of machine learning.
Lastly, the ability to store and process all this data is a crucial factor. Cloud storage and computing have experienced dramatic growth recently, enabling lower costs and higher performance. Additionally, Graphic Processing Units (GPUs) have become faster and cheaper. GPUs are often used with AI because they excel at performing numerous simple calculations concurrently. A modern GPU can handle ten thousand simple calculations concurrently, whereas a modern CPU may only be able to handle a few.
In summary, today’s AI expansion is the culmination of vast amounts of data, freely shared ML techniques, and low-cost, ample cloud storage, and GPU-driven processing.

Figure 2. Example V/TI Monitor Cluster exception that contains a combination of multiple exception types [2]
Limitations of AI
As previously mentioned, a key aspect of a well-performing AI tool is having broad and exhaustive training data. While all the aforementioned AI tools are progressively improving with each new release, there are still situations where they exhibit limitation and inaccuracies. These AI errors are often referred to as “hallucinations,” where the AI tool confidently provides an incorrect answer. An example would be an LLM citing a reference that doesn’t exist or an AI-generated image with an incorrect number of fingers for a person. With these hallucinations, a human expert still needs to be in the loop to identify and ensure that these errors are not incorporated into the final product. Generally, AI tools are more prone to hallucinations when they lack sufficient training data.
For the rail industry, much of its information is not publicly available or is obscure. For example, there are several orders of magnitude more Calico cats’ photographs on the internet than there are of No. 20 turnouts. This means that there was more training data for cat photos than turnout photos, resulting in a relatively poorer AI tool for railway applications and more prone to hallucinations.
Lastly, there are legal considerations. Because the AI tools utilize training data from other sources, it has raised the question, “who owns the output?” There is no clear answer, and each AI tool provides its own usage disclaimers, ranging from the outputs being publicly owned to the prompter being the owner. For any user of AI tools, it is important to understand the tool’s ownership stance and adhere accordingly.
AI in the Rail Industry
The recent AI tools leveraging publicly available data are poised to have a significant impact on industries such as entertainment, art, legal, and news. But what about the rail industry? These AI tools have already assumed an assistant role for many railway professionals, facilitating tasks like proofreading paragraphs or transferring information from PDFs to Excel sheets. Like numerous other industries, the rail sector stands to gain the greatest value from AI tools when its own proprietary data is utilized as training data. Organizations are already exploring in-house versions of AI tools for this purpose. Additionally, the mantra of “Big Data” remains more relevant than ever, with railways prioritizing the retention and storage of data for future AI applications. An application of AI tools likely to impact the rail industry is Robotic Process Automation (RPA), which applies AI automation to office and IT routine tasks.
ML algorithms are versatile and can be applied to various data types, not limited to text and photographs. It can be applied to track geometry data, locomotive event recorder data, train control log files, etc.… An example of this was discussed in the RT&S March 2023 issue where AI was used to predict future track geometry data.[1] This type of AI is particularly useful for the rail industry for decision-support through data analytics. One of the first examples of this was the V/TI Monitor Cluster algorithm. When V/TI Monitors were first deployed, they generated a lot of data, much of which was low-level, non-actionable data. This data was stored for years until the question got asked, “what V/TI data existed at a track-caused derailment site before the derailment?” Leveraging the mountains of V/TI data, that question was answered. In many instances, there was repeated, low-level V/TI activity prior to a derailment occurring as shown in Figure 2.[2] A ML algorithm was built and deployed to identify this pattern and report it to field personnel. In the AREMA paper “V/TI Monitor Cluster Analysis and Implementation”, it was discovered that after the V/TI Cluster exceptions were deployed to field personnel to act on, there was a significant decrease in track caused derailments as shown in Figure 3.[2] Now in this new age of AI, there are more similar opportunities to leverage the latest in analytics to further decrease derailment incidents.
Railway operations, characterized by predictable systems and extensive data collection, lend themselves to the use of “Digital Twins.” These digital replicas of physical systems enable better understanding and facilitate “what-if” analysis, including simulations for derailment investigations. Notably, much of the data used in these simulations existed prior to the derailment, presenting an opportunity to leverage automated digital twins for predictive railway operations.

Figure 3. Effect of implementing the V/TI Monitor Cluster algorithm on reducing derailments [2]
Conclusions
AI will continue to advance significantly in the coming years, ushering in a myriad of applications across various areas of the rail industry. While its impact may not be a tidal wave, the sector can expect a substantial influx of AI applications. The Transportation Technology Center remains committed to advancing safety, streamlining processes, enhancing security, and promoting sustainability within the rail community. Through collaborative efforts with railways, suppliers, universities, and government organizations, the TTC will continue to address testing and research needs, leveraging technology to meet the industry’s evolving goals. Notably, the TTC’s test tracks serve as an ideal location for ground truth testing, ensuring known true-positive conditions are verified and retested for technological and AI advancements.
References
[1] https://issuu.com/railwaytrackstructures/docs/rts_march_2023
[2] D. Clark, M.G. Dick, R.K. Maldonado “V/TI Monitor Cluster Analysis and Implementation” Proceedings of the 2015 AREMA Annual Conference, 2015